Awful gated modelβ¦
raincandy_U
raincandy-u
AI & ML interests
εΉ»θ¦γ
Recent Activity
liked
a dataset
6 days ago
open-thoughts/OpenThoughts2-1M
liked
a dataset
6 days ago
HuggingFaceFW/fineweb-2
liked
a model
6 days ago
google/gemma-3-4b-it
Organizations
raincandy-u's activity
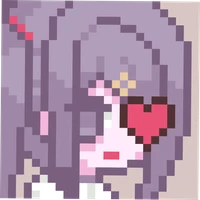
reacted to
beomi's
post with π
6 months ago
Post
7144
# PyTorch == 2.5.0 Breaks Transformers' SDPAttention!
When you encounter "RuntimeError: cuDNN Frontend error: [cudnn_frontend] Error: No execution plans support the graph."
We can use workaround like this:
but this slow downs the performance gain from PyTorch 2.5.
Although it is fixed(not "fixed" but default option is turn-off the cuDNN SDPA) at here -- https://github.com/pytorch/pytorch/pull/138587 , but not released yet. (you need to install directly from source)
Fastest way for now : pip install "torch<2.5"
Ref: https://github.com/huggingface/diffusers/issues/9704#issuecomment-2422585273
When you encounter "RuntimeError: cuDNN Frontend error: [cudnn_frontend] Error: No execution plans support the graph."
We can use workaround like this:
torch.backends.cuda.enable_cudnn_sdp(False)
but this slow downs the performance gain from PyTorch 2.5.
Although it is fixed(not "fixed" but default option is turn-off the cuDNN SDPA) at here -- https://github.com/pytorch/pytorch/pull/138587 , but not released yet. (you need to install directly from source)
Fastest way for now : pip install "torch<2.5"
Ref: https://github.com/huggingface/diffusers/issues/9704#issuecomment-2422585273
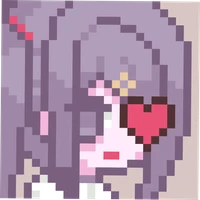
replied to
takeraparterer's
post
6 months ago
What base model are you using?
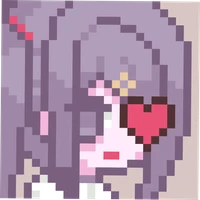
reacted to
takeraparterer's
post with π
6 months ago
Post
2308
Check this out: I trained an AI on huggingface posts! all of these are AI generated:
----------
Hello!
I'm excited to share that my colleague @felipeebert and I have released the largest Spanish LLM benchmark to date.
We've developed the Spanish LLM Evaluation Benchmark (SLAB), a set of benchmarks designed to evaluate the ability of language models to understand, generate and translate in Spanish.
SLAB includes five different benchmarks:
- Sentiment Analysis: evaluate models' ability to detect and describe sentiment in natural language
- Fact Checking: evaluate models' ability to detect and refute factual errors in text
- Question Answering: evaluate models' ability to answer questions in Spanish
- Open-ended Questions: evaluate models' ability to generate coherent responses in Spanish
- Translation: evaluate models' ability to translate in Spanish
SLAB is aligned with the latest Spanish LLM industry developments and includes the most recent models available on the market. We aim to keep our benchmarks up-to-date and relevant to the Spanish language ecosystem.
SLAB is available at: https://huggingface.co/datasets/argilla/SLAB.
If you would like to collaborate on building additional Spanish LLM benchmarks, let's discuss in the comments.
π SLAB Blog Post: https://argilla.com/blog/slab
----------
Hello everyone,
I'm thrilled to announce the release of
https://huggingface.co/01-AI/01AI-GPT-4o -
A new family of models that brings the power of transformer AI to the masses.
This model is designed to be accessible and easy to use, while still offering high-quality results.
Key features:
- Small model size: only 23M parameters
- Supports text generation, image generation, and text-to-image tasks
- Data-efficient training with a lightweight tokenizer
- Optimized for efficient on-device usage
- Uses the powerful transformer architecture to deliver high-quality results
Excited to see what you all think!
https://huggingface.co/01-AI/01AI-GPT-4o
----------
Hello!
I'm excited to share that my colleague @felipeebert and I have released the largest Spanish LLM benchmark to date.
We've developed the Spanish LLM Evaluation Benchmark (SLAB), a set of benchmarks designed to evaluate the ability of language models to understand, generate and translate in Spanish.
SLAB includes five different benchmarks:
- Sentiment Analysis: evaluate models' ability to detect and describe sentiment in natural language
- Fact Checking: evaluate models' ability to detect and refute factual errors in text
- Question Answering: evaluate models' ability to answer questions in Spanish
- Open-ended Questions: evaluate models' ability to generate coherent responses in Spanish
- Translation: evaluate models' ability to translate in Spanish
SLAB is aligned with the latest Spanish LLM industry developments and includes the most recent models available on the market. We aim to keep our benchmarks up-to-date and relevant to the Spanish language ecosystem.
SLAB is available at: https://huggingface.co/datasets/argilla/SLAB.
If you would like to collaborate on building additional Spanish LLM benchmarks, let's discuss in the comments.
π SLAB Blog Post: https://argilla.com/blog/slab
----------
Hello everyone,
I'm thrilled to announce the release of
https://huggingface.co/01-AI/01AI-GPT-4o -
A new family of models that brings the power of transformer AI to the masses.
This model is designed to be accessible and easy to use, while still offering high-quality results.
Key features:
- Small model size: only 23M parameters
- Supports text generation, image generation, and text-to-image tasks
- Data-efficient training with a lightweight tokenizer
- Optimized for efficient on-device usage
- Uses the powerful transformer architecture to deliver high-quality results
Excited to see what you all think!
https://huggingface.co/01-AI/01AI-GPT-4o
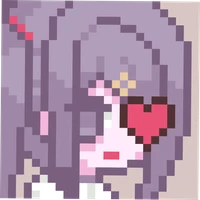
reacted to
zamal's
post with π₯
6 months ago
Post
2091
Hello, lovely community! π
zamal/Molmo-4bit Thrilled to announce that the Molmo 7B 4-bit Space is now live! π The model size has been reduced by six times with almost no performance loss, and the results will leave you amazed!
It runs on zero GPU, making it incredibly accessible for everyone!
Check it out here and start exploring today!
Happy experimenting! π
zamal/Molmo-4bit Thrilled to announce that the Molmo 7B 4-bit Space is now live! π The model size has been reduced by six times with almost no performance loss, and the results will leave you amazed!
It runs on zero GPU, making it incredibly accessible for everyone!
Check it out here and start exploring today!
Happy experimenting! π
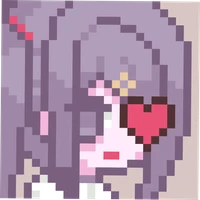
reacted to
Draichi's
post with π€
11 months ago
Post
2289
Hey Hugging Face Community π€
I'm excited to share my latest project that combines my passion for deep learning and racing cars. I recently created a simple method to predict Formula 1 lap times using machine learning . This is the first solution of its kind in the open-source community, and I'm thrilled to present it to you all.
ποΈ The project leverages historical telemetry data to predict lap times, providing a new tool for race strategy and performance analysis. You can check out the notebook on Kaggle here https://www.kaggle.com/code/lucasdraichi/hamilton-lap-time-prediction and see the detailed breakdown of the model and its predictions.
I invite you all to take a look at the lap time predictor, provide feedback, and join the discussion. Your insights and participation would be invaluable as we continue to develop and enhance these tools.
Let's push the boundaries of what's possible with AI in motorsports together!
I'm excited to share my latest project that combines my passion for deep learning and racing cars. I recently created a simple method to predict Formula 1 lap times using machine learning . This is the first solution of its kind in the open-source community, and I'm thrilled to present it to you all.
ποΈ The project leverages historical telemetry data to predict lap times, providing a new tool for race strategy and performance analysis. You can check out the notebook on Kaggle here https://www.kaggle.com/code/lucasdraichi/hamilton-lap-time-prediction and see the detailed breakdown of the model and its predictions.
I invite you all to take a look at the lap time predictor, provide feedback, and join the discussion. Your insights and participation would be invaluable as we continue to develop and enhance these tools.
Let's push the boundaries of what's possible with AI in motorsports together!
Post
2447
π€ I trained what is probably the smallest (600k ~) TinyStories model! It really can write grammatically correct stories!
raincandy-u/TinyStories-656K
Try this space based on this minuscule model!
raincandy-u/Story-Teller
Edit: Moreover, the model weight size is only 1.31MB under bf16, and can be reduced to the 700KB level when using Q8_0 quantization Uβ’γ§β’*U
Edit: Now 1000K params chat model!
raincandy-u/TinyChat-1776K
raincandy-u/TinyStories-656K
Try this space based on this minuscule model!
raincandy-u/Story-Teller
Edit: Moreover, the model weight size is only 1.31MB under bf16, and can be reduced to the 700KB level when using Q8_0 quantization Uβ’γ§β’*U
Edit: Now 1000K params chat model!
raincandy-u/TinyChat-1776K
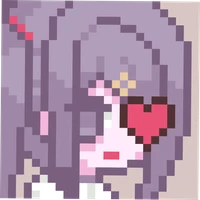
replied to
their
post
11 months ago
Post
2447
π€ I trained what is probably the smallest (600k ~) TinyStories model! It really can write grammatically correct stories!
raincandy-u/TinyStories-656K
Try this space based on this minuscule model!
raincandy-u/Story-Teller
Edit: Moreover, the model weight size is only 1.31MB under bf16, and can be reduced to the 700KB level when using Q8_0 quantization Uβ’γ§β’*U
Edit: Now 1000K params chat model!
raincandy-u/TinyChat-1776K
raincandy-u/TinyStories-656K
Try this space based on this minuscule model!
raincandy-u/Story-Teller
Edit: Moreover, the model weight size is only 1.31MB under bf16, and can be reduced to the 700KB level when using Q8_0 quantization Uβ’γ§β’*U
Edit: Now 1000K params chat model!
raincandy-u/TinyChat-1776K
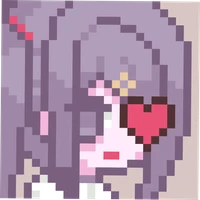
posted
an
update
11 months ago
Post
2447
π€ I trained what is probably the smallest (600k ~) TinyStories model! It really can write grammatically correct stories!
raincandy-u/TinyStories-656K
Try this space based on this minuscule model!
raincandy-u/Story-Teller
Edit: Moreover, the model weight size is only 1.31MB under bf16, and can be reduced to the 700KB level when using Q8_0 quantization Uβ’γ§β’*U
Edit: Now 1000K params chat model!
raincandy-u/TinyChat-1776K
raincandy-u/TinyStories-656K
Try this space based on this minuscule model!
raincandy-u/Story-Teller
Edit: Moreover, the model weight size is only 1.31MB under bf16, and can be reduced to the 700KB level when using Q8_0 quantization Uβ’γ§β’*U
Edit: Now 1000K params chat model!
raincandy-u/TinyChat-1776K
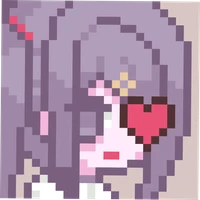
reacted to
mmhamdy's
post with π
11 months ago
Post
1508
π‘ Thinking Tokens For Language Models!
How much is 56 times 37? Can you answer that right away?
In a short paper, David Herel and Tomas Mikolov propose a simple method to improve the reasoning of language models when performing complex calculations.
π They note that, although language models are not that good with difficult calculations, humans also cannot perform these calculations immediately and require a considerable amount of time to come up with an answer.
Inspired by this, they introduce π‘Thinking Tokensπ‘
So what are those "thinking tokens"?! Nothing fancy, they are just special tokens '<T>' that you insert after each word in a sentence whenever a complex problem is encountered. That's it!
π The main idea is to "buy" the model "some time" to think about the problem with these additional computations before answering. Using this method they observed an improved (a little bit) perplexity.
π Before getting excited note that: They have added these tokens manually, and they have used an RNN language model. From the paper:
"As a proof of concept, we have added N βthinking tokensβ (< T >) after each observed word in a dataset. Our vision is that this basic concept can be extended to a self-adjusting model, which will be able to decide itself if and how many βthinking tokensβ will be used for a specific problem, where N could also vary throughout the sentence. This would allow us to reduce the computational time, which would not increase N times."
How much is 56 times 37? Can you answer that right away?
In a short paper, David Herel and Tomas Mikolov propose a simple method to improve the reasoning of language models when performing complex calculations.
π They note that, although language models are not that good with difficult calculations, humans also cannot perform these calculations immediately and require a considerable amount of time to come up with an answer.
Inspired by this, they introduce π‘Thinking Tokensπ‘
So what are those "thinking tokens"?! Nothing fancy, they are just special tokens '<T>' that you insert after each word in a sentence whenever a complex problem is encountered. That's it!
π The main idea is to "buy" the model "some time" to think about the problem with these additional computations before answering. Using this method they observed an improved (a little bit) perplexity.
π Before getting excited note that: They have added these tokens manually, and they have used an RNN language model. From the paper:
"As a proof of concept, we have added N βthinking tokensβ (< T >) after each observed word in a dataset. Our vision is that this basic concept can be extended to a self-adjusting model, which will be able to decide itself if and how many βthinking tokensβ will be used for a specific problem, where N could also vary throughout the sentence. This would allow us to reduce the computational time, which would not increase N times."
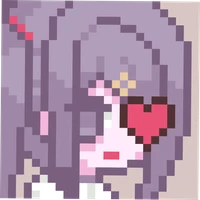
reacted to
zamal's
post with ππ₯
12 months ago
Post
1327
Finally!
My first post for the lovely community out there!
Here's a highly quantized finetuned version of gemma focused exclusively on Prompt Engineering. Write as ambiguous you want and leave the job to this model
zamal/gemma-7b-finetuned
My first post for the lovely community out there!
Here's a highly quantized finetuned version of gemma focused exclusively on Prompt Engineering. Write as ambiguous you want and leave the job to this model
zamal/gemma-7b-finetuned
Post
1976
First post, thanks HF! π€
Here is a Claude 3 Sonnet generated dataset using prompts from WildChat:
raincandy-u/claudy-chat-5k
Here is a Claude 3 Sonnet generated dataset using prompts from WildChat:
raincandy-u/claudy-chat-5k
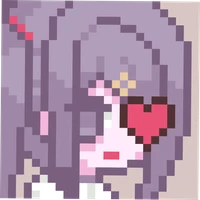
posted
an
update
12 months ago
Post
1976
First post, thanks HF! π€
Here is a Claude 3 Sonnet generated dataset using prompts from WildChat:
raincandy-u/claudy-chat-5k
Here is a Claude 3 Sonnet generated dataset using prompts from WildChat:
raincandy-u/claudy-chat-5k
It will produce repeated output