VisualCloze: A Universal Image Generation Framework via Visual In-Context Learning (Implementation with Diffusers)
Note: You still need to install our modified version of diffusers.
[Paper] β [Project Page] β [Github]
[π€ Online Demo] β [π€ Dataset Card]
π Key Features
An in-context learning based universal image generation framework.
- Support various in-domain tasks.
- Generalize to unseen tasks through in-context learning.
- Unify multiple tasks into one step and generate both target image and intermediate results.
- Support reverse-engineering a set of conditions from a target image.
π₯ Examples are shown in the project page.
π§ Installation
Install diffusers from our modified repository.
git clone https://github.com/lzyhha/diffusers
cd diffusers
pip install -v -e .
π» Diffusers Usage
Example with Depth-to-Image:

import torch
from diffusers import VisualClozePipeline
from diffusers.utils import load_image
# Load in-context images (make sure the paths are correct and accessible)
image_paths = [
# in-context examples
[
load_image('https://github.com/lzyhha/VisualCloze/raw/main/examples/examples/93bc1c43af2d6c91ac2fc966bf7725a2/93bc1c43af2d6c91ac2fc966bf7725a2_depth-anything-v2_Large.jpg'),
load_image('https://github.com/lzyhha/VisualCloze/raw/main/examples/examples/93bc1c43af2d6c91ac2fc966bf7725a2/93bc1c43af2d6c91ac2fc966bf7725a2.jpg'),
],
# query with the target image
[
load_image('https://github.com/lzyhha/VisualCloze/raw/main/examples/examples/79f2ee632f1be3ad64210a641c4e201b/79f2ee632f1be3ad64210a641c4e201b_depth-anything-v2_Large.jpg'),
None, # No image needed for the query in this case
],
]
# Task and content prompt
task_prompt = "Each row outlines a logical process, starting from [IMAGE1] gray-based depth map with detailed object contours, to achieve [IMAGE2] an image with flawless clarity."
content_prompt = """A serene portrait of a young woman with long dark hair, wearing a beige dress with intricate
gold embroidery, standing in a softly lit room. She holds a large bouquet of pale pink roses in a black box,
positioned in the center of the frame. The background features a tall green plant to the left and a framed artwork
on the wall to the right. A window on the left allows natural light to gently illuminate the scene.
The woman gazes down at the bouquet with a calm expression. Soft natural lighting, warm color palette,
high contrast, photorealistic, intimate, elegant, visually balanced, serene atmosphere."""
# Load the VisualClozePipeline
pipe = VisualClozePipeline.from_pretrained("VisualCloze/VisualClozePipeline-384", torch_dtype=torch.bfloat16)
pipe.enable_model_cpu_offload() # Save some VRAM by offloading the model to CPU
# Run the pipeline
image_result = pipe(
task_prompt=task_prompt,
content_prompt=content_prompt,
image=image_paths,
upsampling_width=1024,
upsampling_height=1024,
upsampling_strength=0.4,
guidance_scale=30,
num_inference_steps=30,
max_sequence_length=512,
generator=torch.Generator("cpu").manual_seed(0)
).images[0][0]
# Save the resulting image
image_result.save("visualcloze.png")
Example with Virtual Try-On:
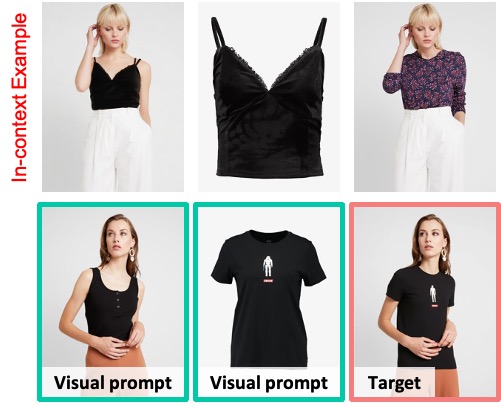
import torch
from diffusers import VisualClozePipeline
from diffusers.utils import load_image
# Load in-context images (make sure the paths are correct and accessible)
image_paths = [
# in-context examples
[
load_image('https://github.com/lzyhha/VisualCloze/raw/main/examples/examples/tryon/00700_00.jpg'),
load_image('https://github.com/lzyhha/VisualCloze/raw/main/examples/examples/tryon/03673_00.jpg'),
load_image('https://github.com/lzyhha/VisualCloze/raw/main/examples/examples/tryon/00700_00_tryon_catvton_0.jpg'),
],
# query with the target image
[
load_image('https://github.com/lzyhha/VisualCloze/raw/main/examples/examples/tryon/00555_00.jpg'),
load_image('https://github.com/lzyhha/VisualCloze/raw/main/examples/examples/tryon/12265_00.jpg'),
None
],
]
# Task and content prompt
task_prompt = "Each row shows a virtual try-on process that aims to put [IMAGE2] the clothing onto [IMAGE1] the person, producing [IMAGE3] the person wearing the new clothing."
content_prompt = None
# Load the VisualClozePipeline
pipe = VisualClozePipeline.from_pretrained("VisualCloze/VisualClozePipeline-384", torch_dtype=torch.bfloat16)
pipe.enable_model_cpu_offload() # Save some VRAM by offloading the model to CPU
# Run the pipeline
image_result = pipe(
task_prompt=task_prompt,
content_prompt=content_prompt,
image=image_paths,
upsampling_height=1632,
upsampling_width=1232,
upsampling_strength=0.3,
guidance_scale=30,
num_inference_steps=30,
max_sequence_length=512,
generator=torch.Generator("cpu").manual_seed(0)
).images[0][0]
# Save the resulting image
image_result.save("visualcloze.png")
Citation
If you find VisualCloze useful for your research and applications, please cite using this BibTeX:
@article{li2025visualcloze,
title={VisualCloze: A Universal Image Generation Framework via Visual In-Context Learning},
author={Li, Zhong-Yu and Du, Ruoyi and Yan, Juncheng and Zhuo, Le and Li, Zhen and Gao, Peng and Ma, Zhanyu and Cheng, Ming-Ming},
journal={arXiv preprint arXiv:2504.07960},
year={2025}
}
- Downloads last month
- 76
Inference Providers
NEW
This model isn't deployed by any Inference Provider.
π
Ask for provider support
Model tree for VisualCloze/VisualClozePipeline-384
Base model
black-forest-labs/FLUX.1-Fill-dev